The concept of Rational agent in AI in the 1950s, with the development of early AI models the Logic Theorist and the General Problem Solver. Systems aimed to mimic human problem-solving processes by using logical reasoning and rule-based approaches. Over the years, advancements in AI algorithms and computational power have contributed to the development of more sophisticated rational agents capable of performing complex tasks.

What is Rational Agent in AI?
Let’s understand what is Rational agent in AI. it refers to an autonomous entity that can perceive its environment, reason about it, and take actions to achieve specific goals. Unlike traditional AI systems that rely on pre-programmed rules, for instance rational agents have the ability to make decisions based on their understanding of the world and optimize their actions to achieve optimal outcomes.
Rationality in AI Systems
Rationality plays a crucial role in the design and implementation of AI systems. By enabling agents to reason and make rational decisions, AI systems can effectively navigate dynamic and uncertain environments. Moreover, this ability to exhibit rational behavior allows AI systems to adapt to changing circumstances, optimize resource allocation, and achieve desired outcomes in a wide range of domains, from autonomous vehicles to medical diagnosis.
Characteristics of Rational Agent in AI
Sensory Input in Rational Agents
- Firstly, Perception forms the foundation of a rational agent’s understanding of its environment. Through sensory inputs, rational agents gather information about the state of the world and interpret it to make informed decisions.
- Sensory inputs include visual data from cameras, audio data from microphones, or even data from specialized sensors radar or lidar.
- By continuously perceiving and updating their representations of the environment, rational agents can make more accurate assessments and predictions.
Importance of Perception in Decision-Making
- Perception is crucial for rational agents as it allows them to gather relevant and up-to-date information about their surroundings.
- This information forms the basis for the decision-making process, enabling agents to assess the current state of the world and choose appropriate actions to achieve their goals. Without accurate perception, rational agents would struggle to make informed decisions, potentially leading to undesirable outcomes or even failure.
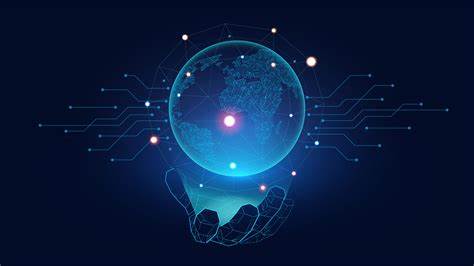
Applications of Sensing in AI
- The application of sensing in AI systems is vast and varied. In autonomous vehicles, for example, sensors such as cameras, lidar, and radar enable the vehicle to perceive its surroundings and make real-time decisions regarding acceleration, braking, and steering.
- In healthcare, sensors can be used to monitor patients and provide real-time feedback, allowing for more accurate diagnoses and personalized treatment plans. Overall, sensing plays a crucial role in enabling rational agents to interact with their environment and make intelligent decisions.
Knowledge and Reasoning
It involves the process of encoding information about the world in a format that utilized by the AI system. Likewise, this representation can take various forms, such as logical rules, ontologies, or probabilistic models. By effectively representing knowledge, rational agents can reason about the world, acquire new information, and make informed decisions based on their understanding.
Types of Reasoning in AI Systems
Rational agents employ different types of reasoning to process and manipulate their knowledge. Deductive reasoning, for example, involves deriving conclusions from general principles or rules. Inductive reasoning, on the other hand, involves making generalizations based on observed patterns. Additionally, abductive reasoning allows rational agents to infer the most likely explanation for a given set of observations. By combining these reasoning mechanisms, rational agents can effectively analyze complex situations and make optimal decisions.
The Role of Logic in Rational Agent’s Decision-Making
Logic plays a significant role in the decision-making process of rational agent in AI. By utilizing logical rules and formal reasoning systems, rational agents can evaluate the consequences of their actions, maintain consistency in their knowledge representation, and derive logical inferences. Logic provides a structured framework for rational agents to reason and make decisions, ensuring that their actions align with logical principles and objectives.
Decision-Making and Planning
The Decision-Making Process in Rational Agents
- Decision-making is an essential aspect of rational agent in AI. It involves selecting the most appropriate actions or plans to achieve a particular goal. Rational agents use their perception, knowledge, and reasoning capabilities to assess the available options, evaluate their potential outcomes, and determine the optimal course of action.
- The decision-making process of rational agents is dynamic and iterative, with agents continuously monitoring the environment and adapting their decisions as new information becomes available.
Importance of Planning in Rational Agent’s Actions
- Planning plays a crucial role in the actions of rational agents in AI. By engaging in the process of planning, rational agents can anticipate future states, analyze potential courses of action, and create a sequence of actions to achieve their goals. Planning allows rational agents to consider long-term consequences, allocate resources effectively, and mitigate risks associated with their actions.
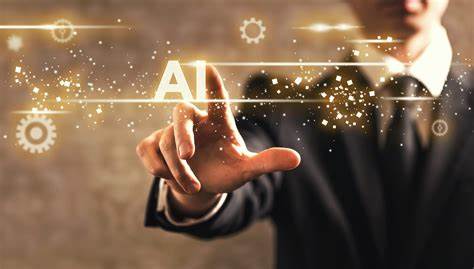
Rational Agents and Learning
The Role of Learning the Rational Agent in AI
Learning is an integral component of rational agent in AI. It enables agents to acquire new knowledge, adjust their behavior based on experience, and optimize their decision-making processes. Learning in AI systems can take various forms, including supervised learning, unsupervised learning, and reinforcement learning. By incorporating learning mechanisms, rational agents can adapt to changing environments, discover patterns, and improve their performance over time.
Types of Learning in AI Systems
AI systems employ different types of learning to enhance the capabilities of rational agents. Supervised learning involves training an agent using labeled examples, enabling it to make predictions or classifications based on previous observations. on the other hand, focuses on discovering patterns or structures in unlabeled data, enabling rational agents to uncover hidden insights. Reinforcement learning leverages a reward system to guide agents’ actions, allowing them to learn optimal behavior through trial and error.
Benefits and Limitations of Learning the Rational Agent in AI
- Learning provides several benefits to rational agent in AI. It allows to adapt the new situations, improve their performance over time, and handle complex tasks that cannot be pre-programmed. Learning also enables rational agents to generalize from past experiences, making them more flexible and robust.
- However, learning also has its limitations. similarly, It can be time-consuming, computationally intensive, and may require a considerable amount of training data. Moreover, learning models are susceptible to biases and may struggle when faced with rare or novel situations.
Reinforcement Learning and the Rational Agent in AI
- Reinforcement learning, a subfield of machine learning, is particularly relevant to the rational agent framework. By optimizing their actions to maximize rewards over time, rational agents can learn optimal policies for achieving their goals.
- Reinforcement learning found applications in various domains, for example robotics, game playing, and autonomous systems, enabling rational agents to learn complex behaviors and strategies.
Balancing Exploration and Exploitation
Exploration vs. Exploitation Dilemma in AI
- One of the key challenges in the design of rational agents is striking a balance between exploration and exploitation. Exploration refers to the act of gathering new information or trying out new actions, while exploitation focuses on maximizing short-term rewards based on existing knowledge.
- Rational agents need to explore their environment to discover new opportunities and update their knowledge, similarly they also need to exploit their current knowledge to make optimal decisions. Finding the right balance between exploration and exploitation is crucial for the long-term performance of rational agents.
Improving Decision-Making through Exploration
- Exploration in rational agents is not merely a means to gather new information; and most importantly improving in decision-making. By exploring new possibilities, rational agents can discover more optimal solutions or uncover hidden patterns in the environment. Exploration allows rational agents to escape local optima, avoid biases,
- And continuously improve their decision-making processes. certainly, through a balanced exploration and exploitation strategy, rational agents can not only achieve short-term goals but also optimize their long-term performance in dynamic and uncertain environments.
Transparency in rational agent in AI
- In order to build trust and ensure the ethical use of AI systems, however it is crucial that rational agents’ decision-making processes are transparent and understandable.
- Transparency allows users and stakeholders to understand the reasoning behind the decisions made by rational agents, enabling them to evaluate the system’s behavior and identify potential biases or errors. above all by providing transparency, rational agents can enhance their accountability and facilitate human oversight and intervention when necessary.
Explaining AI Decisions to Humans
- Above all the challenges in the development of rational agents is explaining their decisions to humans in a transparent and interpretable manner. and it Explain ability ensures that the decision-making processes of rational agents can be understood and scrutinized by humans.
- Techniques such as rule extraction, attention mechanisms, and surrogate models have been proposed to provide explanations for AI decisions. By bridging the gap between the internal workings of rational agents and human understanding, to clarify AI promote trust, facilitate collaboration between humans and AI systems, and ensure ethical decision-making.
Balancing Transparency and Confidentiality
- Secondly transparency is crucial, it must be balanced with concerns of confidentiality and privacy. Rational agents may process sensitive or personal data, necessitating the protection of individuals’ privacy rights.
- Also, Developers establish mechanisms to maintain the confidentiality of sensitive information still providing sufficient transparency to address concerns related to bias, fairness, and accountability.
- Striking the right balance between transparency and confidentiality ensures that rational agents operate ethically and responsibly without compromising individuals’ privacy.
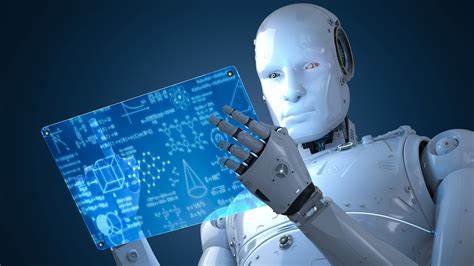
Ethical Considerations in Rational Agents
Bias and Fairness in AI Systems
- Recognizing and addressing bias in rational agent in AI is a critical ethical consideration. If not properly addressed, AI systems perpetuate societal biases and discrimination. Developers ensure that rational agents are trained on unbiased, diverse datasets and thoroughly evaluate their decision-making processes to mitigate the potential for biased outcomes.
- Similarly, Fairness and justice should be prioritized, guaranteeing that rational agents make decisions that align with ethical standards and to sum up do not discriminate against individuals or groups based on race, gender, or other protected characteristics.
Conclusion
In short, the rational agent in AI model provides a conceptual lens through which we can analyze and design intelligent systems. It encapsulates the essence of AI—creating agents that can perceive, reason, and act in ways that lead to optimal outcomes. As a result, at this point AI continues to advance, refining and expanding the rational agent in AI framework will remain integral to the development of more sophisticated and capable artificial intelligences.
Recommended Reads:
Top Machine Learning Courses in Kolkata
Top 8 Artificial Intelligence Courses in Pune
16 Best Nanotechnology Books to read in 2023.
Top 10 Machine Learning Courses in Mumbai
AI in Manufacturing: Here is All You Need to Know
FQAs
Q.1: What is Rational agent in AI?
in short, it refers to an autonomous entity that can perceive its environment, reason about it, and take actions to achieve specific goals. Unlike traditional AI systems that rely on pre-programmed rules, for instance rational agents have the ability to make decisions based on their understanding of the world and optimize their actions to achieve optimal outcomes.
Q.2: What are some real-world applications of rational agent in AI?
Rational agents find applications in various fields, including robotics, autonomous vehicles, game playing, finance, healthcare, and more. In each of these domains, rational agents are employed to make decisions and take actions to achieve specific goals.
Q.3: Can a rational agent adapt to changes in its environment?
Yes, additionally a rational agent is often designed to be adaptive. This adaptability can involve learning from experience, updating beliefs, and adjusting strategies over time. It allows the agent to respond effectively to changes in its environment.
Q.4: Can a rational agent learn from experience?
Yes, after all many rational agents are designed to learn from experience. This involves updating their knowledge and adapting their decision-making strategies based on the outcomes of past actions.
Q.5: What are the key characteristics of a rational agent?
Key characteristics include being goal-oriented, processing information from the environment, making decisions based on goals and current knowledge, adaptability to changes, autonomous operation, and managing uncertainty.